Deep-learning based multi-scale computational ghost imaging for high-performance complex image recovery
点击次数:
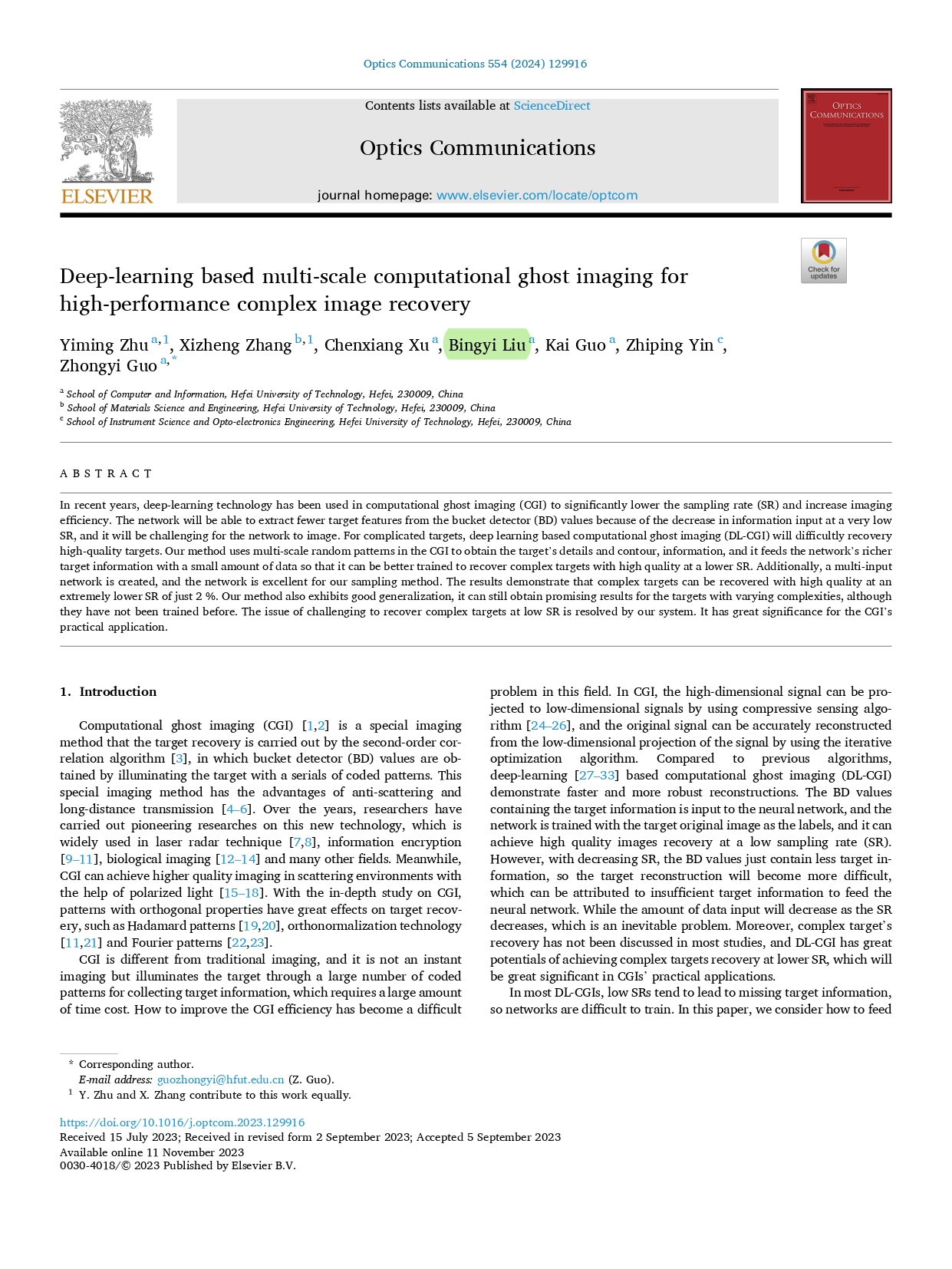
DOI码:10.1016/j.optcom.2023.129916
发表刊物:Optics Communications
摘要:In recent years, deep-learning technology has been used in computational ghost imaging (CGI) to significantly lower the sampling rate (SR) and increase imaging efficiency. The network will be able to extract fewer target features from the bucket detector (BD) values because of the decrease in information input at a very low SR, and it will be challenging for the network to image. For complicated targets, deep learning based computational ghost imaging (DL-CGI) will difficultly recovery high-quality targets. Our method uses multi-scale random patterns in the CGI to obtain the target's details and contour, information, and it feeds the network's richer target information with a small amount of data so that it can be better trained to recover complex targets with high quality at a lower SR. Additionally, a multi-input network is created, and the network is excellent for our sampling method. The results demonstrate that complex targets can be recovered with high quality at an extremely lower SR of just 2 %. Our method also exhibits good generalization, it can still obtain promising results for the targets with varying complexities, although they have not been trained before. The issue of challenging to recover complex targets at low SR is resolved by our system. It has great significance for the CGI's practical application.
合写作者:Xizheng Zhang,Chenxiang Xu,Bingyi Liu,Kai Guo,Zhiping Yin
第一作者:Yiming Zhu
论文类型:期刊论文
通讯作者:Zhongyi Guo
文献类型:J
卷号:554
期号:129916
是否译文:否
发表时间:2023-12-06
收录刊物:SCI