Point Cloud-based Optimization of Roadside LiDAR Placement at Constructed Highways
点击次数:
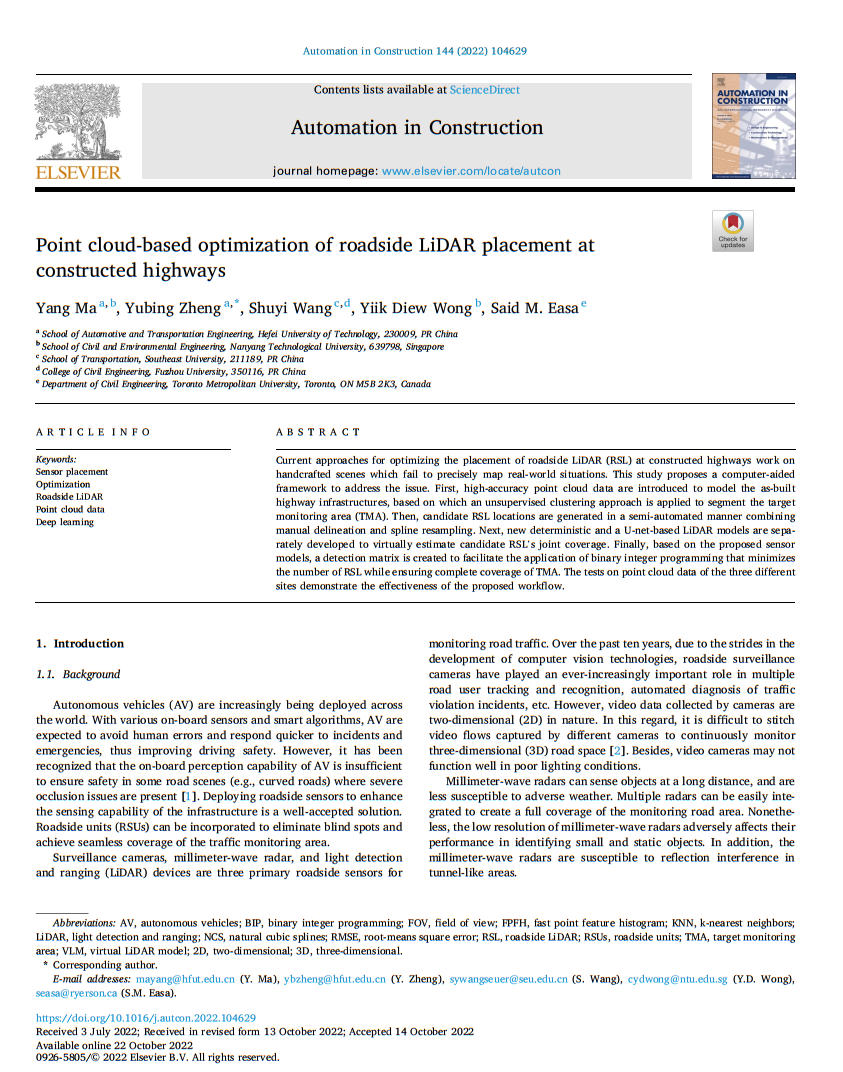
影响因子:10.517
DOI码:10.1016/j.autcon.2022.104629
所属单位:汽车与交通工程学院
发表刊物:Automation in Construction
刊物所在地:Netherland
项目来源:合肥工业大学人才引进基金;加拿大自然科学与工程研究基金
关键字:Sensor placement, Optimization, Roadside LiDAR, Point cloud data, Deep learning
摘要:Current approaches for optimizing the placement of roadside LiDAR (RSL) at constructed highways work on handcrafted scenes which fail to precisely map real-world situations. This study proposes a computer-aided framework to address the issue. First, high-accuracy point cloud data are introduced to model the as-built highway infrastructures, based on which an unsupervised clustering approach is applied to segment the target monitoring area (TMA). Then, candidate RSL locations are generated in a semi-automated manner combining manual delineation and spline resampling. Next, new deterministic and a U-net-based LiDAR models are separately developed to virtually estimate candidate RSL’s joint coverage. Finally, based on the proposed sensor models, a detection matrix is created to facilitate the application of binary integer programming that minimizes the number of RSL while ensuring complete coverage of TMA. The tests on point cloud data of the three different sites demonstrate the effectiveness of the proposed workflow
备注:智慧建造Top期刊
合写作者:王书易,Wong, Yiik Diew,Easa, Said
第一作者:马羊
论文类型:期刊论文
通讯作者:郑玉冰
论文编号:104629
学科门类:工学
文献类型:J
卷号:144
期号:2022
页面范围:104629
字数:10946
是否译文:否
发表时间:2022-10-18
收录刊物:SCI、EI
发布期刊链接:https://www.sciencedirect.com/science/article/pii/S092658052200499X
附件:
Point cloud-based optimization of roadside LiDAR placement at constructed highways.pdf